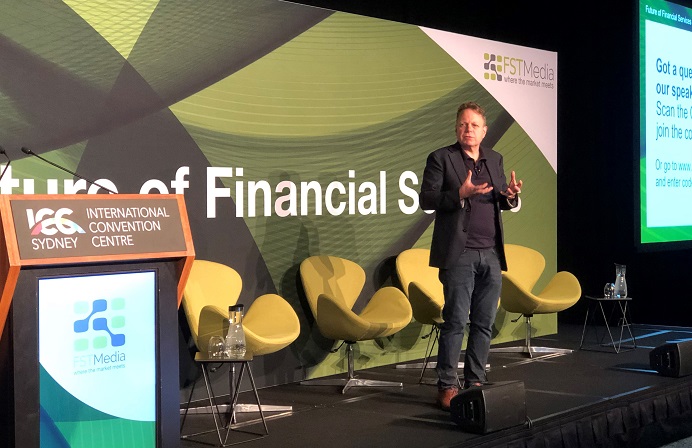
ANZ Bank is experimenting with multiple Generative Artificial Intelligence (GenAI) technologies, including an in-house developed ChatGPT client that will eventually be used to train customer-serving banking staff, says chief technology officer Tim Hogarth.
The platform, christened by ANZ techs as ‘Z-GPT’, was developed internally by the bank’s engineering team and based on an engine similar to that which underpins the publicly accessible ChatGPT language model.
Z-GPT is categorised by ANZ as a ‘knowledge base’ bot, specialised to input and distil information from the bank’s publicly available dataset – a particularly challenging prospect for any corporate with a sprawling catalogue of information, Hogarth noted.
“We all know what it’s like trying to find information in a corporate network – it’s really, really difficult. And perfect taxonomies don’t exist, so we have to search.”
Z-GPT is among a number of workforce ‘augmentation’ bots being developed and tested by ANZ’s engineering team, including dedicated platforms for document authoring and analysis, as well as coder assistance tools to support programmers.
As well, Hogarth said, the bank is also experimenting with a GenAI bot being developed “with another partner”.
“These are novel and will materially change the way our workforce operates,” Hogarth said, speaking at the Future of Financial Services Sydney, 2023 conference. “We will realise significant improvements in productivity, quality or throughput.”
“But the [one] we’re actually quite proud of, and one of the things we’re looking at right now is Z-GPT.”
According to Hogarth, Z-GPT functions as a ‘private instance’, meaning its large language model (LLM) is isolated from the public domain and restricted to ANZ’s enclave, “trained and tuned” to the bank’s dataset.
“This is similar to the public GPT… but it’s private, which means we can use internal information and do things a little more safely.”
The LLM scrubs data from publicly accessible ANZ domains (ANZ.com.au), including bluenotes – a large repository of news, research and opinions created by ANZ and external contributors – among others.
“What this [bot] allows is to determine questions to, say, ‘What’s the interest rate that ANZ Plus [ANZ’s dedicated digital banking arm] pays on deposit accounts?’.
“[Z-GPT] then searches and frames that question, narrowing it down to just the context that’s on the ANZ.com.au site, and then comes back with a very precise answer: ‘ANZ Plus pays 4.65 per cent on balances below $250,000’.
“The [platform] can also very quickly tell us that, once you tip over that amount, the amount you get is 1.5 per cent,” Hogarth said.
While this is a very straightforward interaction, he said, this question can also be posed in “fairly casual language”, with the bot utilising advanced natural language processing – for instance, ‘What happens if I’ve got a lot more money than that?’ or ‘What if I have waaaaay more moolah than 250 grand?’

ANZ’s own ‘Chat-GPT’ play – Z-GPT
Welcoming the use of an open-source GPT, Hogarth admitted ANZ’s tech team would “never be able to build a large language model from scratch that is this accurate.”
He confirmed that ANZ’s current incarnations of GenAI will likely never be seen by customers.
“We’re keeping this just for ourselves,” Hogarth said.
“This is just something we’re using internally to [determine whether] it produces reliable results.”
He added, however, that the tech team plans to deploy the technology for its bankers to support their customer service role and to “see if they can find answers more rapidly and reliably”.
For Hogarth, more than simply an augmentation tool for staff, AI is fundamentally transformative for the industry, particularly in reshaping how consumers interact with their banks.
“This technology would be able to meld traditional bank data with any other data, giving the customer significant insight into their finances.”

ANZ’s experiments with AI
He added: “This is about language. It’s about converting this language to that language. It’s converting this specification, to this piece of code, to that website. Or, it’s taking these receipts, turning it into an expense language and then comparing it against a policy.”
“Now I can ask, ‘What is the total expense on these 16 receipts and does it match this policy?’.
“We’ve never been able to do that ever before in the history of business or banking.”