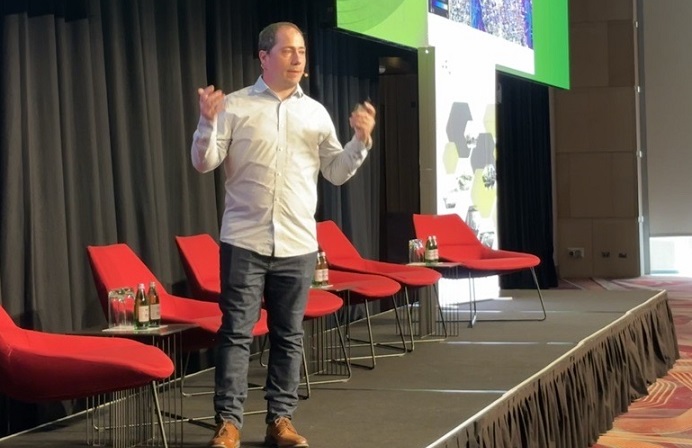
Generative artificial intelligence (Gen AI) represents a step-change development for the insurance sector and will significantly enhance insurers’ business operations, with QBE Ventures’ global head of emerging technology Alex Taylor urging insurers to start experimenting with the technology now before competitors get the upper hand.
“I’ve lived hype cycles,” Taylor said during his keynote presentation at FST’s Future of Insurance 2024 conference, noting his co-founding of a Bitcoin company in the 2010s.
“I’ve seen interest wane in a space when the gravity of what something might do evaporates into a lack of enthusiasm when it becomes evident that something doesn’t work.
“If there’s one thing that I’d love to collectively [impart to you] today is that AI is not a fad. It’s not something that will go away. And this is particularly so for Generative AI, which represents such an advance in our industry… [that] it will never be the same,” he said.
“This is a space that’s not just hype and froth, and bubble. It is something that has real impact.”
Already, incumbent global insurers, including AXA, Chubb, RGA and Lloyds are beginning to operationalise Gen AI technologies in-house, including dedicated rollouts of GPT and other large language model (LLM) functions.
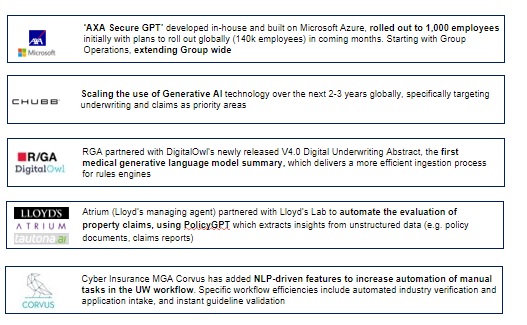
Taylor notes global insurers active in generative AI
Insurers must brace for change. For an industry seldom accustomed to moving quickly, Taylor acknowledged, the “accelerative capability” of Gen AI technologies will either force insurers to adopt and adapt, or leave them trailing – potentially forever – behind.
“While we’re long been used to being able to deploy a system and confidently say that’s what’s going to be running for the next 20 years, that’s not the case anymore.”
Echoing analysts’ predictions, Taylor said it will be at least five years to a decade before AI technologies can be trusted to do “exactly what a human would do”. However, the exponential advance of AI over just the last two years, from the “clever parlour tricks” of early ChatGPT to today answering real-world questions, and the diminishing cost of using these services presents a clarion call to action for all industries.
The gateway for insurers into Generative AI, or large language model (LLM), technologies will likely be in ‘retrieval augmented generation’. In layman’s terms: document data extraction. Effectively, this enables users to extract via a natural language prompt relevant information from a piece of media content, whether it be text, image, audio or video.
“[Retrieval augmentation] is the space that is going to accelerate the operations that we have today,” Taylor said.
Taylor cited a use case applied in Google’s experimental Generative AI studio, Gemini 1.5 Pro, which applies natural language and hand-drawn prompts to extract precise information (see below image) from old films. For insurers, such technology could be used to extract relevant data from long-form documents or to examine photo evidence presented within claims.

Google’s Gemini 1.5 Pro asked by a human to pinpoint when “a piece of paper was removed from a person’s pocket” in a Buster Keaton film
“The kinds of tasks that we can use this [technology] for in insurance, particularly broker-led insurance or claims operations, are where you need to know something quickly and don’t have deeper information to find out, or if you want to relate information together, its utilities are even more strongly enhanced.
Whilst fears abound that these technologies will lead to mass human redundancies, Taylor argued this is not the case, instead serving to augment existing human capabilities often wasted on menial tasks.
“Generative AI is faster and better than we are as humans at ingesting and understanding and considering vast amounts of information in parallel,” he said.
“That one thing – document analysis – by itself is disruptive enough that a lot of operations that are done today manually by people are vastly accelerated by this particular capability.”
For instance, he recognised AI’s ability to cut down the time it takes to rate the Environmental, Social, and Governance (ESG) credentials of a company (noting its use by QBE for portfolio optimisation) from a full day with a human at the helm to just seconds with a Gen AI model.
“Reading hundreds of pages of documentation as complex as numbers as fast as big as the images is hard, and it’s expensive to do. And if you want to do it over a large portfolio of companies, it takes a long time.
“A machine doing the same task takes about 20 seconds. And you can ask all of the questions in parallel and get the result in front of you… in a structured format, and ready for inserting into a database.
“The key theme is acceleration. This is not replacing jobs; it’s helping people to do tasks that are done today – very laboriously, very manually – extremely quickly. And to get to that point of value where a human can contribute much, much faster.”
Multimodal language models in insurance
Gen AI, of course, has applications far beyond document analysis, with Taylor noting various use case experiments by QBE Ventures, the global insurer’s venture investment and development arm.
Gen AI has become progressively multimodal, meaning that it can accept inputs of text, images, audio, and video for contextual and reasoned analysis of these media “in parallel”. Moreover, it is accessible to those without a programming or deep technology background.
Taylor cited the use of a ChatGPT-style Gen AI model to analyse satellite imagery for the purposes of claims or underwriting risk assessment – for example, assessing ‘before and after’ bird’s eye imagery to assess damage from a cyclone or other natural disaster. Traditionally, this would require either a human or a trained in-house computer model (developed by an insurer at the cost of “many millions”) to analyse.
“Now, anybody can just do this [analysis] through ChatGPT,” Taylor said.
“And I can tell you right now that if we ask the model to give us responses in a structured format, this is where the technologists may start to think, ‘Hey, I can put this straight into a database that can do eFNOL… or [request additional] human oversight’.
“Being able to do this kind of task where we see a building that we care about and want to underwrite, we want to raise a claim on and being able to give us a prompt like this – this is an example of ‘prompt engineering’.
“But people are evolving their use of them rapidly because you don’t need to be an engineer to do this anymore. Trial and error from non-technical people or business stakeholders that write things like this becomes very powerful.”
Existing AI-based technologies are also set to be turbocharged by these large language models.
Taylor cited the work of Geosite, a core investment for QBE Ventures, which enables insurers to assess changes in environments, and thus insurable property assets, based on satellite-imaged maps.
The technology makes extensive use of synthetic aperture radar (SAR), a laser-guided system that can map “within a couple of centimetres” the distance between the satellite and the ground, as well as other ground-based geospatial data.
It can track any changes within a particular region – from natural disaster movements or damages to property, environmental or property risk analyses, or property valuations – to evaluate a premium or even activate a claim.
“It would have taken a couple of months [before] to actually get around to visiting all of those properties with a notebook and pen to figure out what’s taking place or to start to activate supply chains.
“Now we can do things like look at the fact that there are solar panels on the roof of this building… to start securing the supply chain to do that remediation.”
Building a Generative AI strategy
For Taylor, despite the importance of tech teams and strategists driving Generative AI innovation, any Gen AI strategy will only get off the ground with buy-in from the executive team.
“Understanding what will be disrupted and over what time period is essential,” Taylor said.
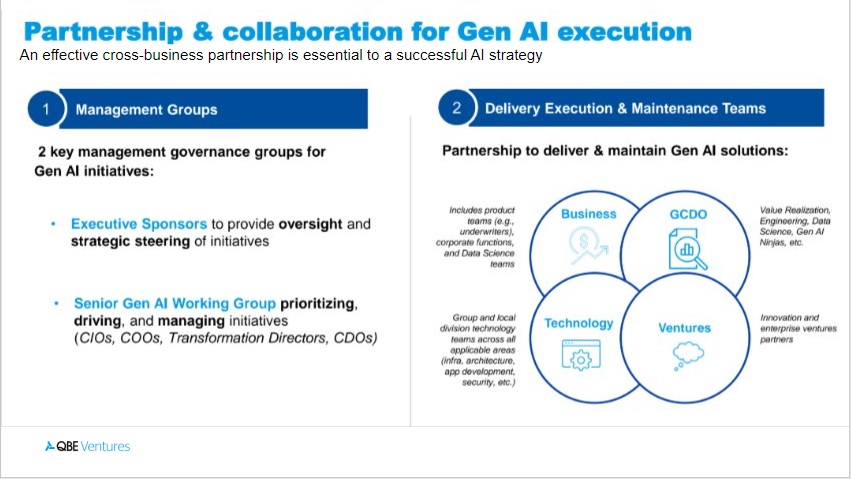
Taylor’s ‘high-level’ generative AI strategy
“You need to bring in the best and brightest of your organisation together, from data from business teams – and business teams have so much value to add in a world of Generative AI systems that you don’t need to be a machine learning engineer to interact with.”