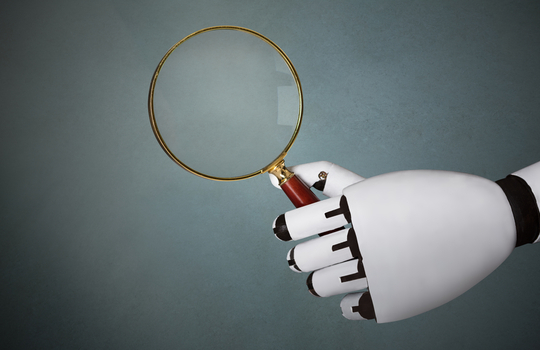
Machine learning offers “the greatest advantage” to financial services in tackling money laundering and terrorism financing risks through existing systems, a new report by the global AML/CTF watchdog has found. However, organisations must be conscious of “bad data” that could bedevil AI/ML models, potentially creating more problems than it solves.
The Financial Action Task Force’s (FATF) Opportunities and Challenges of New Technologies for AML/CTF report – which comes off the back of a FATF global survey of industry stakeholders – also cited distributed ledger technology (DLT), or blockchain, natural language processing, and APIs, alongside machine learning (ML), as crucial technologies in the fight against money laundering and terrorism financing (ML/TF).
The report noted that machine learning (ML) systems offer financial services unique advantages through their ability to learn from existing systems, reducing the need for manual input for AML/CTF monitoring, reducing false positives, and identifying complex cases, as well as facilitating risk management.
It stressed, in particular, the considerable value of ML systems in supporting remote customer onboarding and verification, enabling “microexpression analysis, anti-spoofing checks, fake image detection, and human face attributes analysis”.
Using behavioural and transactional analysis, ML algorithms can also “monitor and [give] alert scorings… on patterns of activity”, ensuring transaction anomalies can quickly be tracked and mapped.
Utilising an ‘unsupervised ML algorithm’ could, furthermore, help to cluster customers “into cohesive groupings based on their behaviours”, creating risk-based controls that can tailor and provide more “efficient monitoring of the business relationship”.
Organisations can also leverage the technology to rapidly adapt to evolving regulations. ML can serve to identify and implement regulatory updates, scanning and interpreting large volumes of unstructured regulatory data sources on an ongoing basis “to automatically identify, analyse and then shortlist applicable requirements for the institution” that boost compliance.
ML can also be supplemented with complementary natural language processing (NLP), cognitive computing capabilities, and robotic process automation (RPA) to support this regulatory compliance process.
AI-backed NLP, which simulates human decision-making – taking “incomplete, ambiguous, distorted, or inaccurate (fuzzy) inputs to produce useful outputs” – can also serve FSIs as part of its broader AML/CTF monitoring systems to identify specific alerts or areas of higher risk.
NLP, crucially, helps regulated entities and the watchdogs themselves bridge data quality gaps, “as the programmes become better at linking elements of information”, for instance, “connecting search engine results with PEP lists, identifying fraud attempts, monitoring sanctions lists”.
The report made reference to the Italian Financial Intelligence Unit’s (UIF) use of fuzzy logic to devise AML indicators for non-banking financial intermediaries. The technology has ensured quantitative data (i.e. cross-border payments from/to higher risk countries) could be “elaborated” to support periodic AML/CFT risk assessment of intermediaries.
“The source of data used for computing the indicators is the aggregate anti-money-laundering reports… database and supervisory reports.”
The FAFT however cautioned that adoption of machine learning and other data-hungry technologies for AML/CTF functions need to be carefully managed, being particularly careful not to feed in bad data that could create more problems than it solves.
Indeed, the capacity to leverage new technologies for AML/CFT will, it stressed, only be effective if systems are based on “standardised data that is easier for technology developers to integrate into their tools, easy to understand and explain to non-experts, and easy to communicate to counterparts and competent authorities when needed”.
It added that while new technologies are no doubt improving data quality, machine-based systems will continue to rely on human input and manual review.
“Machine learning tools rely on existing systems and their manual updating, thus possibly generating instances where ‘bad data’ is inputted and has a negative impact on the models adopted.”
“This includes the data which a machine learning system is trained on, e.g. to learn to identify suspicious transactions.
“If the training data includes false positives or other errors, these errors will be ‘trained-in’ to the machine learning system, although some margin for error will still be needed for instances of human bias or unidentified errors.”
Other technologies in the mix
Blockchain, or DLT, was also recognised as offering significant value in for AML/CTF fight, improving traceability of cross-border transactions – not just locally, but “on a global scale” – and simplifying identity verification.
DLT, the report noted, “may speed up the customer due diligence process”, enabling consumers to essentially “authenticate themselves”.
“They can even be automatically approved or denied through smart contracts that verify the data.”
However, it cautioned that DLT may, due to its uniquely decentralised structure, “continue to pose challenges from an AML/CFT perspective… [that] enable un-intermediated peer-to-peer transactions without any scrutiny”.
Use of application programming interfaces or APIs, the report noted, could also offer significant advantages to augment AML/CTF systems, particularly in improving interoperability between banking and regulatory systems.
APIs were widely recognised by survey respondents as the “most used and relevant solutions to the identified money laundering and terrorist financing problems”, FATF said.
“Their utility for AML/CFT lies in the ability to, for example, connect customer identification software with monitoring tools, or risk and threats identification tools with customer risk profiles in order to generate alerts or alter risk classifications as relevant.
“APIs allow this integration to happen much more quickly and with much larger datasets.”
API’s, it added, “also offer great value to the public sector by helping them access business registries and others, and providing the “agility to be modified for temporary monitoring purposes in response to unexpected shocks to the economy or more permanently in response to changes in financial system business models.”
The FATF, however, noted that adoption and implementation of new AML/CFT technologies has been stymied, for the most part, by either regulatory or operational hurdles.
“Data collected for this report suggest that there is a need for clear support from FATF and national competent authorities for innovation in AML/CFT.”
A few experts expressed a desire to have “technology-active supervisors” – supervisors willing to engage with technology developers – rather than technology-neutral approaches.
“Respondents believe that a lack of express support by competent authorities and FATF has led to diminished interest, investment and trust in new technologies, despite their potential.”
The UN’s Office on Drugs and Crime estimates that the amount of money laundered globally in a single year equates to between two and five per cent of global GDP – or between $ 1.1 trillion to $US2.7 trillion.