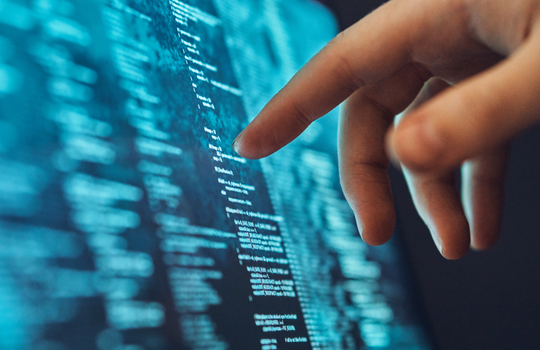
The Australian Human Rights Commission (AHRC) has raised concerns around potentially unlawful discrimination risks arising from Artificial Intelligence (AI), with tech chiefs from financial services also stepping up to root out errors in AI-backed customer systems, as tackling algorithmic bias tops global FSI data leaders’ post-pandemic priorities.
A new paper by the AHRC, co-published with the Gradient Institute, Consumer Policy Research Centre, CHOICE and the CSIRO’s Data 61, has warned companies, including financial institutions, that the use of predictive AI systems compromised by algorithmic bias could lead to breaches of existing anti-discrimination laws.
Algorithmic bias is a systematic error in AI systems, typically within Machine Learning (ML) models, stemming from inaccuracies in datasets or flaws in a model’s programming, whereby predictive outcomes either create or perpetuate social inequalities.
The AHRC paper outlined a particular case study where a hypothetical energy retailer uses AI to identify a segment of ‘profitable customers’ – that is, customers that bring a net positive value to the business – that are deemed to be worth offering more competitive rates to.
Using a simulated dataset, the paper showed how a predictive AI system could inadvertently produce discriminatory selections against women, Indigenous Australians and older people, thus contravening existing anti-discrimination and equal opportunity laws.
For example, a historical bias in a dataset involving women’s earnings could lead to lower-than-actual estimations of women’s ‘profitability’, thus disadvantaging female customers when obtaining a service contract.
This could, in effect, contravene Australia’s Sex Discrimination Act 1984 or corresponding state or territory law, according to the AHRC.
“Because algorithmic bias can result in unlawful activity, there is a legal imperative to address this risk,” said Ed Santow, Human Rights Commissioner.
“However,” he stressed, “good businesses go further than the bare minimum legal requirements to ensure they always act ethically and do not jeopardise their good name”.
For FSIs, the paper warned, algorithmic bias could trickle into unfair customer outcomes in mortgage or credit card applications, other forms of credit decisioning, as well as risk underwriting for insurers.
The AHRC’s findings were echoed in large part by Shameek Kundu, chief data officer at the Singapore-based Standard Chartered Bank, during his keynote address at FST’s Future of Financial Services, Sydney 2020 conference earlier this month.
Affirming AHRC’s views, Kundu believed that AI’s promise to augment enterprise performance would be hollow unless financial firms proactively address the technology’s considerable risks, particularly in algorithmic bias.
“Perhaps the most dangerous or difficult situation [with AI usage] is the unjust bias challenge, where machine learning models are at greatest risk of reinforcing biases,” Kundu said.
In Kundu’s view, data bias has always existed, even with traditional statistical analytical models.
However, as uptake of AI/ML technologies advances across the enterprise – while, at the same time, human intervention is gradually reduced – the potential for algorithmic bias is one leaders can no longer ignore.
For Kundu, there is no “silver bullet” to address these biases. However, a grounding, industry-wide code could serve as an important basis for principled innovation.
“There’s a lot of work to be done in terms of sharing external and internal best practices… right now, they’re just good practices – emerging good practices – through communities of practice inside the bank, plus with other organisations,” he said, alluding to FSIs’ ad-hoc, self-regulatory approach to the technology.
The issues surrounding ethics and AI bias are set to dominate financial services’ technology agendas for the foreseeable future, he said.
Fellow Future 2020 speaker Darren Klein, general manager of data and analytics at MLC Life, noted that potential sources of bias in AI will “go up… not down” in a post-Covid economy, where structural shifts are aplenty. This could lead to more imperfect datasets and, therefore, a greater likelihood of model drifts that compromise AI’s decision-making capability.
In a post-pandemic economy, propped by the government’s business-recovery 2020 Budget, Klein flagged ongoing changes around consumer spending and wealth creation, swings in the labour market, as well as global supply chains.
These market disruptions, Klein said, combined with growing cost pressures, will shine new light on the importance of data accuracy, model reliability, as well as greater AI uptake to boost efficiency at scale.
Klein urged industry to “pay more attention” to algorithmic biases, considering the risks that compromised (and historically inaccurate) predictive models could introduce to customer service delivery and outcomes.
“At the moment, we have regulation at every layer – the Consumer Data Right, privacy data laws, and even the regulation of digital platforms. But at the moment, there are no regulations for algorithms or AI themselves,” he added, echoing Kundu’s sentiments.
“As an industry… we do need to move from [being] ethical to accountable in AI usage.”
“There is a question about whether moving from ethical to accountable is actually a pipe dream, or if it’s just a matter of time,” he added, acknowledging the considerable opportunities AI presents for FSIs.
Making the most of AI’s potential
Australian financial firms, according to Klein, have historically placed AI development within digital teams in order to support customer journey development – for instance, enabling customers to see real-time insights on their finances through a mobile app.
Going forward, however, Klein anticipates a growing trend where standalone AI capabilities will be established to address increasing back-office needs (primarily through increased automation and process optimisation), in response to post-pandemic cost pressures.
Standard Chartered’s Kundu, for one, is scaling AI initiatives across the bank. However, he stressed the need to balance rapid uptake of the technology with appropriate risk management, including a focus on educating the global bank’s 85,000-strong staff – many of whose roles are, or will be, impacted by AI technologies.
Apart from identifying key sources of algorithmic bias (including different base rates, historical bias, label bias, contextual features and under-representation of groups, as well as inflexible models), AHRC’s technical paper also proposed five mitigation strategies.
These are: acquiring up-to-date and more comprehensive data points; pre-processing data before using it to train an algorithm; programming an AI system to identify nuanced differences between groups; modifying the AI system to correct for existing inequalities; and finding fairer measures to target variables when prompting the AI to make assessments.
Kundu similarly mapped out key risk areas specific to AI/ML adoption, including the explainability and reliability of a model’s results, stability of models in a changing economic climate, auditability of models, plus, data bias and real-world social biases that FSIs must be wary of.
“[AI] is not just an opportunity. It’s an imperative for traditional banks,” he said.
“This is not just because it’s the secret to profitability or lower cost… but because it’s the only way banks can meet their core purpose of serving customers across all parts of society in a fair and responsible way.”
Kundu further emphasised the proactive steps made by financial regulators in Singapore and Hong Kong – markets where Standard Chartered has a significant presence – in publishing guidelines to tackle AI bias.
Closer to home, while Data61 released an economy-wide AI roadmap late last year setting out eight high-level principles for ethical AI use, as yet no FSI-specific guidelines from regulators have been formed.
Where guiding principles are proving helpful, Kundu believes it would be “premature” to introduce new legislation for industry to combat algorithmic bias, citing the nascent nature of AI technology and its yet-to-be-fully understood social impacts.
For now, he said, “I think we have enough safeguards in existing legislation or regulations [in place].”