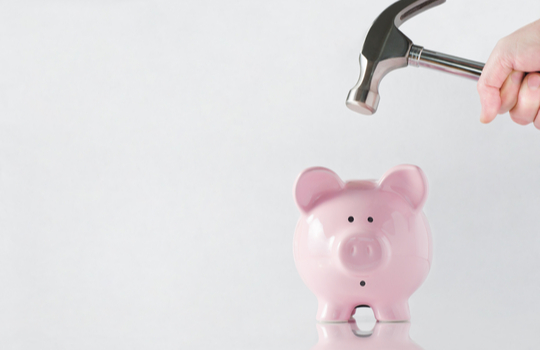
The NSW Ombudsman has called for greater scrutiny and transparency around the use of artificial intelligence (AI) and machine learning (ML) technologies within government following revelations the state’s debt-collection agency, Revenue NSW, had deployed automated technology unlawfully to recover money from bank accounts of debtors to the state (for unpaid fines).
In a report tabled to NSW parliament, the state Ombudsman cautioned agencies that the adoption of machine technologies “in ways that do not accord with standards of lawfulness, transparency, fairness and accountability”, could result in findings of maladministration or potentially unlawful conduct.
The report was triggered after the Ombudsman received a spate of complaints from individuals whose bank accounts had been “stripped of funds and sometimes completely emptied”, according to NSW Ombudsman Paul Miller. Many of the impacted individuals were also deemed to be financially vulnerable.
A number of complainants were welfare recipients whose bank accounts had held funds received from state welfare agency Centrelink as their only source of income, the report revealed. Most appeared unaware that the process of reclaiming debt was automated.
“Those people were not complaining to us about the use of automation,” Miller added in a statement “They didn’t even know about it.” It also appears the Ombudsman was also not aware, until its 2019 investigation, that Revenue NSW was using ML for its garnishee processes.
The revenue agency first implemented an automated system to issue garnishee (that is, the debiting of money directly from bank accounts) orders in 2016.
Triggered by earlier concerns flagged by the Ombudsman in 2019 around the use of automation for garnishee orders, Revenue NSW instituted a modification in March of that year to introduce a ‘human-in-the-loop’ who would formally authorise these orders.
The agency believed at the time that the change “would avoid any legal doubt as to the lawful exercise of discretionary power under the Fines Act”.
Garnishee orders are one of a range of civil sanctions available under the NSW Fines Act 1996 to recover outstanding fines debt. The orders can only be issued when a fine defaulter has not engaged with Revenue NSW following several notifications of an outstanding debt.
The number of garnishee orders issued by Revenue NSW has swelled over the last decade – from 6,905 in the 2010-11 financial year to more than 1.6 million in 2018-19. Account holders are not given prior notice of the order.
Seeking external legal advice in the wake of the complaints (after Revenue NSW declined to do so), the Ombudsman found the agency’s use of the original machine learning system between early 2016 and March 2019 – without a human to finalise the execution of garnishee orders – to be unlawful.
This, it said, was “because no authorised person engaged in a mental process of reasoning to reach the state of satisfaction required to issue a garnishee order, and because the discretionary power was not being exercised by the authorised person”.
While Miller acknowledged that Revenue NSW “to its credit… took a number of steps to address our concerns about unfairness [in 2019]”, it did not “seek expert legal advice on whether the use of the automation process was lawful and in accordance with its powers under the Fines Act” despite recommendations from the Ombudsman it do so.
The Ombudsman also warned that the revenue agency’s ‘traffic light system’, adopted in 2019, may also be subject to legal challenges without a statutory amendment or internal policy changes to alter the process.
The traffic light system also uses an automated, business rules-based algorithm to check against ‘inclusion’ and ‘exclusion’ criteria of individuals subject to garnishee orders. Once the 11 ‘inclusion’ criteria and 16 ‘exclusion’ criteria are met (and therefore ‘green lit’ by the system), a human authorises the sending of an electronic order to the banks in the form of a Check Summary Report.
Most of these criteria are based strictly on quantitative conditions (such as whether the defaulter is between the ages of 18 and 70 or registers vulnerability score above 35).
Since September 2018, a machine learning model within the DPR [Debt Profile Report – a business rules engine that generates customer profiling] has also been used to identify and exclude “vulnerable persons” from the application of garnishee order processes.
“If all the traffic lights were green, the reviewer would proceed to approve the making of the garnishee orders without giving any specific consideration to the file of the underlying fine defaulters,” the Ombudsman wrote.
“Absent express statutory amendment, we accordingly do not think that a statutory discretion can be lawfully exercised by giving conclusive effect to the output of an information technology application. “
“We do not think that the unlawfulness is altered by that output being broken down into component parts (i.e. the considerations raised in the Check Summary Report) and the decision-maker proceeding, as matter of course, to exercising the power (i.e. issuing the garnishee orders because all the traffic lights were green) without engaging in a mental process to justify that conclusion.”
The Ombudsman further warned that “[declining] to consider all, or part, of a fine defaulters file would seem to us to carry the risk that the Commissioner might make a garnishee order in circumstances which would be considered be procedural unfair.”
Government agencies are not currently obligated to proactively report on their use of machine technology. Moreover, agencies are not required to inform individuals when decisions affecting them are being made by or with the assistance of machines.
“The use of machine technology in the public sector is increasing, and there are many potential benefits, including in terms of efficiency, accuracy and consistency,” Miller said.
“As an integrity agency, our concern is that agencies act in ways that are lawful, that decisions are made reasonably and transparently, and that individuals are treated fairly.
“Those requirements don’t go away when machines are being used.”
Ombudsman recommendations
The report offers guidance to agencies on reducing the risk that a machine learning technology could be unlawful or lead to maladministration.
These include:
- ensuring the design involves experts from fields other than IT, including legal advisors. Indeed, in 2004, the Administrative Review Council had underscored the need for lawyers to be actively involved in the design of machine technology for government
- giving careful consideration to the relationship between the machine and the ultimate human decision-maker
- building in a rigorous pre-deployment testing and ongoing auditing regime, and
- taking action to ensure appropriate transparency.
“We are concerned that other agencies may also be designing and implementing machine technologies without appreciating all the risks, without transparency, and without getting appropriate legal advice’, Miller said.
The report also suggested that Government agencies consider seeking Parliamentary approval through legislation before machine technology is adopted for important administrative functions.
“Seeking express legislative authorisation not only reduces the risks for agencies,” Miller said, “it also gives Parliament and the public visibility of what is being proposed, and an opportunity to consider what other regulation of the technology may be required.
“That might include a statutory right to ask for a person to review any machine decision, or a requirement that the machine’s algorithms be externally validated, and then audited at regular intervals, with those reports to be made publicly available.”
Following the tabling of the report, the NSW Ombudsman’s Office said it would engage with the NSW Government and local government sector to comprehensively map the use of machine technology in administrative decision-making processes across the state.
“Greater visibility is not a panacea to all of the potential issues that can arise when Government adopts machine technology,” Miller said. “But it is an essential starting point.”