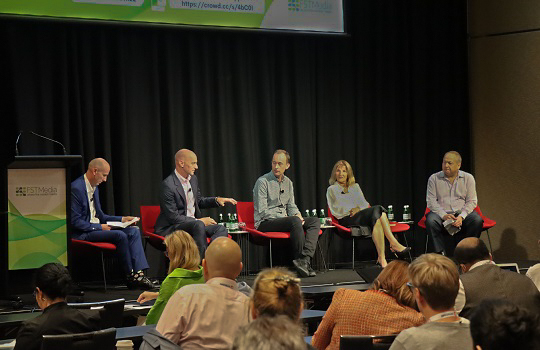
The road to the future of insurance is paved in data. But is the industry laying a solid data foundation or leaving itself a sodden mud path?
We take a peek at some of the highlights from the Advancing Data Analytics in Insurance panel discussion featured at the 2022 Future of Insurance event.
Senior data leaders from Australia’s general and life insurance sectors laid out their roadmaps to AI and machine learning adoption, noting the increasingly vital role of unstructured data and the need for more ‘free play’ with this fast-growing asset, and the radical evolution of the actuary as new data changes the fabric of risk analysis.
Featured panellists:
Katarina Dulanovic, General Manager Data Office, Allianz Australia
Peter Histon, Chief Technology Officer, Resolution Life Australasia
Duncan Cordell, Chief Data and Operations Officer (Life), Munich Reinsurance Australasia
Chris Lee, Senior Manager and Observability Strategist, Splunk
Moderator: Anthony Scott, Chief Executive Officer, Simplyai
Scott (Moderator): Artificial intelligence is made up of two very important pillars of automation and data, which ultimately underpin its success. These enable you to get good customer outcomes like personalisation, claims processing and dynamic pricing.
So, recognising its importance, where are you up to on your AI journey?
Dulanovic (Allianz): That’s a really good question. Allianz is a global organisation and we, like every other big global organisation, have a number of technology solutions in play, from RPA to AI, integrated voice analytics embedded in, for instance, our first notice of loss [capability]. These are all very active across our global organisation, and they’re now being road-mapped in Australia.
The challenge here though with such technology is that we’re a heavily regulated industry, and we have to tailor those offerings that the rest of the world is using to suit our regulators here.
These challenges aren’t just Allianz-specific.
Histon (Resolution Life): We’ve invested quite a bit in both our core data platform, because that’s at the core of analytics workloads, and then on some automation cases that sit on top of that. A couple of examples are around claims triage, so when we get notified of a claim, we’ve got a triage model, which some might know as a ‘random forest model’. We use that to make sure that when a case comes in, it gets allocated to the most appropriate case manager. And we’ve also done quite a bit in automating the income protection benefit payment process as well.
Cordell (Munich Re): Similar to Allianz, we’re global, so we leverage a lot of the technology we use around the world in both our life and non-life segments.
I work specifically around the life side here and what we’re seeing is great progress from our clients, the big insurers in this space, and we’re preparing ourselves for the acceptance of that information. We’re not on that AI journey on that transactional level at the moment, but we’re preparing ourselves for what comes through from that. And we have a global strategy, Ambition 2025, with digitisation a big part of that, so it’s something we live and breathe every day.
Scott (Moderator): And, Scott [Cordell], being part of a reinsurer, you obviously work very closely with the insurers. How do you get to align your strategy to theirs?
Cordell (Munich Re): Conversation, I think, is the best way. We spend a lot of time really trying to understand what they’re doing. These relationships are based on treaties that we have in place, and there are requirements within that. But what we aim to do is to provide a value-add for our clients. We work with them to help identify areas that they want to develop more, and to determine whether we’ve got a solution that we can provide for them or we can also help them develop a solution.
Scott (Moderator): So we’re looking at technologies that underpin machine learning and natural language processing. Chris [Lee], from the vendor perspective, where are you up to with that and what strategies are you seeing emerging in the marketplace?
Lee (Splunk): The first thing you need to recognise when you’re thinking about machine learning is that not everyone’s a data scientist. You’ve got lots of people from operations that need to utilise the data, but they don’t understand what, say, a ‘random forest’ might be.
One of the really strong methodologies to adopt is to provide a curated experience but also still make available that uncurated experience.
Within Splunk, among our service offerings we’ve got a curated experience to look for, say, anomaly detection or for predictive analytics. These are targeted towards operations teams to give them that utility to get that information but also to be told how to do it. At the same time, you still need to have the ability for people to roll their sleeves up and get deep into it with a machine learning toolkit. They want to be able to be free; they want to have access to all of that data, to pull it in from all those different places and just play with it. After all, data is fun!
Cordell (Munich Re): One hundred per cent agree. I say quite often to my teams, ‘Unstructured data is gold‘. There is a place where, if you have that data and you can actually unpack it, challenge it, play with it and put it in different structures, you come up with some real insights that you would not necessarily have got if you’d gone through those very structured datasets. We’re very fortunate, Munich RE has got deep pockets.
One of our teams in Germany has the title of ‘Data Hunters’. It’s literally just 17 guys that sit with our global data and play with it.
And I’ll get an email every now and again asking, ‘I’ve just been looking at this, what do you think of it?’. And we can take that and validate it with our teams, and with our clients and so on.
I agree, you’ve got to have the option of the two. When we’re receiving data from our clients, the structure of the data is really good as well; without that, it makes it very difficult to put it into the systems of record, because we’ve still got to have that regulatory lens as well. But it’s finding the balance, and we’re fortunate we can go more to that unstructured, more investigative, creative side, whereas some of our clients need to be a little bit more structured. It’s not a one-stop-shop; it’s about what’s right for you as an organisation.
Dulanovic (Allianz): Being from a CDO function, yes, it is really important to allow people to play with all this unstructured data and things like social media. But one thing that’s really important is having a single view of your data, whether it’s virtual or physical.
Playing with data in your own little sandpits, in divisionals, in silos, will really not excel your organisation journey to AI and automation and digital.
Cordell (Munich Re): I one hundred per cent agree. The teams that can play around with it need to be ring-fenced in a sandpit. It’s not about giving it to everybody to do.
Histon (Resolution Life): Picking up on a couple of points. Chris [Lee’s] first: Not everybody is a data scientist. Absolutely. We thought quite deliberately about it given our AI agenda, and so we’ve got very capable data scientists who understand what random forest is and what all the other models are and who focus on solving really difficult data science problems.
But we’ve also created, based on our agile structure, a translators’ chapter: they’re people who can communicate and understand business problems but have been through training to understand what the art of the possible is from an AI and advanced analytics point of view.
And that’s been working really well for us, because you’ve sometimes got – in my experience – solutions looking for problems, and this chapter has really helped to bridge that gap between those who establish the business problems that we want to solve and those who look for how we can solve them.
On the point around structured versus unstructured data – both are important. You can learn a whole heap of stuff from unstructured data, but to Duncan [Cordell’s] point, once you need to take that through either a reinsurance purpose or a reporting purpose then that’s completely different and you need that data governance in place to support that. But, of course, it costs more to get it out of your unstructured layer all the way through.
Scott (Moderator): On the point of delighting the customer, the technology to me is fairly straightforward. The biggest struggle organisations have is around change and bringing people along the journey. Chris [Lee], to start with you, how do you support people, often who are scared of what they don’t know?
Lee (Splunk): The hardest part of my career is dealing with people and change. There’s no simple way around it.
Customer experience is key, yet I’m still coming across too many organisations that live in what I term ‘customer-based monitoring’ – waiting for customers to tell them there’s a problem.
Dealing with change and trying to move these big, slow organisations into that customer-focused point of view is imperative. How you do it is, frankly, is through just as Duncan [Cordell] referred to: conversations, education, talking about the art of the possible, what’s actually useful, how you can use that data.
Cordell (Munich Re): People and change… I’d love to know the right answer. Empowering people to actually think about it in a creative way, but to also keep them focused is the balance you need to establish. Not only with our clients but internally people come and say, ‘Oh, the data is going to say X to us’, I need to report on claims’. Well, what questions are you going to ask? What do you need it for? What’s it going to drive? What’s it going to change? And getting people to take that next step in their change journey, asking ‘Why am I doing this?’ instead of just doing it because it’s the in thing. Because once they’ve got there, it’s then very easy to get the tools in place, the mindsets and the processes to deliver on what they’re looking for and provide that rich information.
I spend probably about 45 to 50 per cent of my time on that change on the people side of it.
Some people are very staid as well. We have a very high proportion of actuaries in our business who have been in the market for a long time; they have some really great creative ideas, but then trying to pull them into the modern way of doing things or looking at the tools and saying, ‘Well, we could do it this way!’, that’s always a big challenge.
Lee (Splunk): One of the key parts when you’re trying to get people to ask questions and find answers within the data is to look at the possible first; go right back, before you even look at the data, look at those questions and answers, and then find the data to support it and feed it. Don’t do it the other way around. Otherwise, you go out with this really narrow view.
Histon (Resolution Life):
Some of our best data scientists are, in fact, reformed actuaries.
And I think it’s because they understand what the data means. They’re really great at understanding the data and using it to solve real business problems.
Another thing we did about a year ago is that we invested pretty heavily in a data literacy program, ensuring all of our people could understand what the art of the possible was and why things like data governance and data quality are important. That’s helped us as well.
Dulanovic (Allianz): I wanted to pick up on what was said earlier around people, and it’s really around education; people don’t want to know what these models mean. However, we do need to teach them.
We have a program we’re running at Allianz right now with our risk colleagues, with a really open-minded risk team and leader. This program is around bringing those concepts of models down to the people: What does it mean to have simple models? What is a complex model? What is a deep learning model? Because within our organisations, they don’t all speak the same language.
That education around AI needs to happen as well – across all teams, not just data scientists who should know what they’re doing – and those programs need to be supported by risk teams, because the more we move into AI, the greater the risk. You’ve got simple risk from simple models and you’ve got high risk from when you get into deep learning and then AI. How do you govern that? This is the education that needs to happen now across your organisation to be able to have those conversations in the future as well.
Scott (Moderator): Allianz is about to launch very rapidly into this space and they’re starting in HR. Is that a really good decision, to help that socialisation of technology to support adoption right across the organisation?
Dulanovic (Allianz): To pick up on a point I made earlier, all organisations have different divisions at different levels of maturity in using data, advanced analytics technologies and so forth.
We’re starting off with a use case in HR because they really have advanced in how they use data; there’s a lot of data that HR we can tap into, including from social media. They have got a business problem to solve and we need to start with them. To me, that’s a step up. Once you get from one division that’s really willing, you can then tackle areas that are a little bit harder to move with new technologies. So, I see it as the perfect use case.
Scott (Moderator): In the next five years, where are we going to be at?
Cordell (Munich Re): There’ll be some companies that are using AI and machine learning really, really well, and there’ll be some that will languish, not through any fault of their own but because they’ve perhaps not looked at the structure or prepared for how they’re going to do it up front.
Looking at our clients that are doing a good job of it, they’ve actually stopped and taken the time to review it to ask, ‘What do we want to do?’, ‘What is our aim ten years out from now?’, and they’ve given themselves that space and have got the whole business on-board with it; from there, they’ve then driven it forward as a business-wide piece. I think in five or ten years from now, they’ll be the ones that are market-leading, especially in the life space.
The one thing I would say from the life insurers’ perspective, the big barrier is going to be regulation. If there’s significant change in regulation, for instance, that could slow things down.
Histon (Resolution Life): We spoke about actuaries before, and not to sound crass about it, but what a lot of actuaries do is look at what happened in the past and then provide models that have been around for decades to try to predict what’s happening and what’s going to happen in the future. And in a lot of cases, it’s using Excel and spreadsheets and simply mashing data together.
The actuarial profession I think will completely change and they will become data scientists, because the models are always going to be more efficient than humans.
All of our roles will change as the technology evolves, and those organisations that don’t evolve with the technology will, as you say Duncan [Cordell], probably be left behind.
Cordell (Munich Re): And the strength of that is that people from your claims adjudicators and your underwriters are going to have time to actually think. The tools are going to make things efficient, and they’re going to have time to think about how do they deliver added value. That’s the real benefit of it.
Dulanovic (Allianz): In five years’ time, at least within the general insurance space, our business model will need to change. We’ll need to figure out what, exactly, we are insuring in the future. You’ve got driverless cars coming, you’ve got home devices, and they’re all electronic and talking to each other. We need to ask: Who are we insuring and how are we insuring them?
Based on that, and this is where technology comes in, we really need to harness a lot more Internet of Things data than we’re currently doing.
This is where regulators have to come and play nice with us, because we cannot do that without their support; we can’t grow our industry without their support.
Lee (Splunk): Katarina [Dulanovic] just called out the big point there. The volume of data in the next five to ten years is going to change exponentially, which means the number of people who are looking at that data will also need to change. These teams are going to grow and there’s going to be more information sourced from devices, such as cars, for risk analysis. This sheer wave of information we’ll use in some way, and probably in ways that we haven’t even figured out yet. But, as I said before, data is fun.
This is an edited extract from the Advancing Data Analytics in Insurance panel discussion featured at the Future of Insurance, Sydney 2022 event.