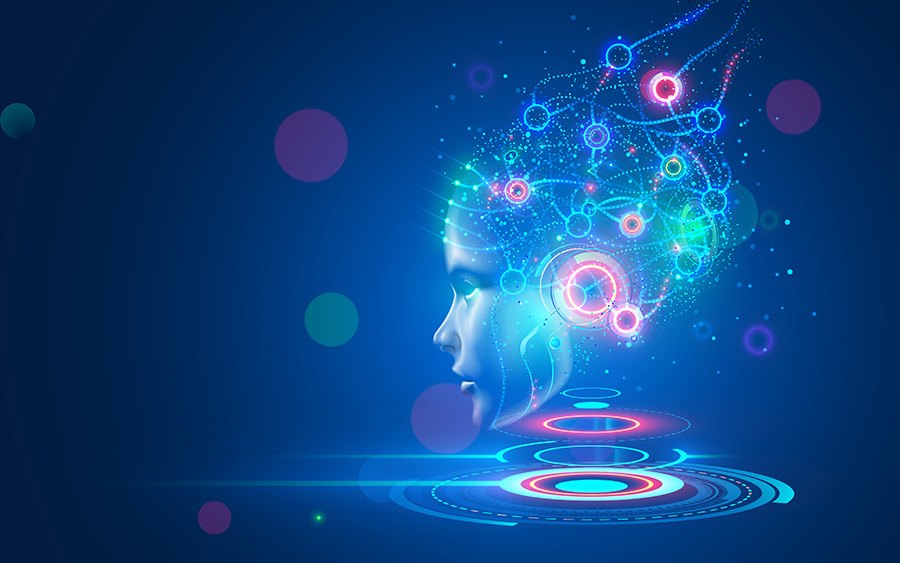
Algorae Pharmaceutical, an Australia-based pharmaceutical development company focused on producing novel medical treatments, has joined as a participant in the CSIRO’s Next Generation Artificial Intelligence (AI) graduate program (NGAIGP) that is hoped to advance AI innovation in pharma sector.
The company, which is listed on both the Australian Securities Exchange (ASX) and in the United States, has said in its announcement that the use of AI in drug discovery and development had the potential to revolutionise the pharmaceutical landscape.
Under the terms of the multi-party agreement, which includes the University of New South Wales, three PhD candidates will be recruited for the purpose of advancing the development of Algorae Operating System. Following this, the candidates will be supervised by AlgoraeOS lead investigator and AI expert, Professor Fatemeh Vafaee and another post-doctoral operating officer.
The hand-selected PhD candidates will also form part of the research and development (R&D) team for the AlgoraeOS project to be co-funded by CSIRO and Algorae. The national science agency will provide approximately two-thirds of the funding required for the candidates, with Algorae kicking in the remaining third over the three-year term of scholarships.
The Next Generation Graduates program is managed by the CSIRO and delivers partnerships with industry and universities to grow a pipeline of home-grown and job-ready graduates, with the hope of unlocking economic opportunities offered by AI as well as other emerging technologies, such as robotics, cyber security, quantum computing and blockchain.
“AI applied to pharmaceuticals also has the potential to streamline research activities, such as preclinical and clinical study design, and facilitates the repurposing of existing drugs, either alone or in combinations, for new therapeutic uses,” the firm said in the announcement to the ASX.
“AI algorithms analyse complex data, such as clinical data, biological data, molecular structures, and genetic information, enabling rapid identification of drug targets with higher precision and efficiency.
“Machine learning, deep learning and neural network models predict drug interactions, assess toxicity, and optimise compound designs, guiding researchers towards more promising avenues of investigation while saving time and resources.”